Databricks Interview Q&A- Part 1
1.How to connect the azure storage account in the Databricks?
To connect the azure blob storage in the databricks, you need to mount the azure stoarge container in the databricks. This needed to be done once only. Once the mounting is done, we can starting access the files from azure blob storage using the mount directory name. For creating the mount you need to provide the SAS token, storage account name and container name.
dbutils.fs.mount(
source = "wasbs://<container-name>@<storage-account-name>.blob.core.windows.net",
mount_point = "/mnt/<mount-name>",
extra_configs = {"<conf-key>":dbutils.secrets.get(scope = "<scope-name>", key = "<key-name>")})
- <container-name> is the name of the container to which you want to connect.
- <storage-account-name> is the name of the storage account which you want to connect.
- <mount-name>is a DBFS path representing where the Blob storage container or a folder inside the container (specified in
source
) will be mounted in DBFS - <conf-key> it can be
fs.azure.account.key.<storage-account-name>.blob.core.windows.net
orfs.azure.sas.<container-name>.<storage-account-name>.blob.core.windows.net
or you can use the secrets from key store
2.How to import third party jars or dependencies in the Databricks?
Go to the cluster and select the libraries. Under the libraries you will see ‘Install new ‘ tab click on it. Now it will ask for the type and source of the library to be installed. Provide the details and click on the Install.
3.How to run the sql query in the python or the scala notebook without using the spark sql?
In the databricks notebook you case use the ‘%sql’ at the start of the any block, that will make the convert the python/scala notebook into the simple sql notebook for that specific block.
4.How many different types of cluster mode available in the Azure Databricks?
Azure Databricks provides the three type of cluster mode :
- Standard Cluster: This is intended for single user. Its can run workloads developed in any language: Python, R, Scala, and SQL.
- High Concurrency Cluster: A High Concurrency cluster is a managed cloud resource. It provide Apache Spark-native fine-grained sharing for maximum resource utilization and minimum query latencies. High Concurrency clusters work only for SQL, Python, and R. The performance and security of High Concurrency clusters is provided by running user code in separate processes, which is not possible in Scala.
- Single Node Cluster: A Single Node cluster has no workers and runs Spark jobs on the driver node.
5.How can you connect your ADB cluster to your favorite IDE (Eclipse, IntelliJ, PyCharm, RStudio, Visual Studio)?
Databricks connect is the way to connect the databricks cluster to local IDE on your local machine. You need to install the dataricks-connect client and then needed the configuration details like ADB url, token etc. Using all these you can configure the local IDE to run and debug the code on the cluster.
pip install -U "databricks-connect==7.3.*" # or X.Y.* to match your cluster version.
databricks-connect configure
6.What are the different pricing tier of the azure databricks available ?
There are basically two tier provided by the azure for databricks service :
7.What is the Job in Databricks?
Job is the way to run the task in non-interactive way in the Databricks. It can be used for the ETL purpose or data analytics task. You can trigger the job by using the UI , command line interface or through the API. You can do following with the Job :
- Create/view/delete the job
- You can do Run job immediately.
- You can schedule the job also.
- You can pass the parameters while running the job to make it dynamic.
- You can set the alerts in the job, so that as soon as the job gets starts, success or failed you can receive the notification in the form of email.
- You can set the number of retry for the failed job and the retry interval.
- While creating the job you can add all the dependencies and can define which cluster to be used for executing the job.
8.How to create DataFrame from CSV file in pySpark?
Simple dataframe creation from csv file
df = spark.read.csv("file_path.csv")
Create dataframe by auto read schema
df= spark.read.format("csv").option("inferSchema", "true").load("file_path.csv")
Create dataframe with header
df= spark.read.format("csv").option("inferSchema", "true").option("header","true").load("file_path.csv")
9.How to create DataFrame from JSON file in pySpark?
Simple dataframe creation from csv file
df = spark.read.json("file_path.json")
Create dataframe by auto read schema
df= spark.read.format("json").option("inferSchema", "true").load("file_path.json")
Create dataframe with header
df= spark.read.format("json").option("inferSchema", "true").option("header","true").load("file_path.json")
10.Why you should define schema up front instead of schema-on-read approach?
It has three benefit as follows :
• You relieve Spark from the onus of inferring data types.
• You prevent Spark from creating a separate job just to read a large portion of your file to ascertain the schema, which for a large data file can be expensive and time-consuming.
• You can detect errors early if data doesn’t match the schema.
11.You can use the spark sql using the ‘spark.sql()’.
We can create the view out of dataframes using the createOrReplaceTempView() function. For example:
df = spark.read.csv('/FileStore/tables/Order-2.csv', header='true', inferSchema='true')
df.createOrReplaceTempView("OrderView")
Now once view has been created on the dataframes then you can write your logic using the spark sql as follows:
df_all = spark.sql("select * from OrderView")
df_all.show()
12.Databricks widgets
Input widgets allow you to add parameters to your notebooks and dashboards. The widget API consists of calls to create various types of input widgets, remove them, and get bound values.
There are 4 types of widgets:
text
: Input a value in a text box.dropdown
: Select a value from a list of provided values.combobox
: Combination of text and dropdown. Select a value from a provided list or input one in the text box.multiselect
: Select one or more values from a list of provided values.
dbutils.widgets.help("dropdown")
Create a simple dropdown widget.
dbutils.widgets.dropdown("state", "CA", ["CA", "IL", "MI", "NY", "OR", "VA"])
You can access the current value of the widget with the call:
dbutils.widgets.get("state")
Finally, you can remove a widget or all widgets in a notebook:
dbutils.widgets.remove("state")dbutils.widgets.removeAll()
13.Ways to modularize or link notebooks
The %run command allows you to include another notebook within a notebook. You can use %run
to modularize your code, When you use %run
, the called notebook is immediately executed and the functions and variables defined in it become available in the calling notebook.
To implement notebook workflows, use the dbutils.notebook.*
methods. Unlike %run
, the dbutils.notebook.run()
method starts a new job to run the notebook.
14. What is different between ADL and BLOB?

15. what is different between Azure data warehouse vs ADL?
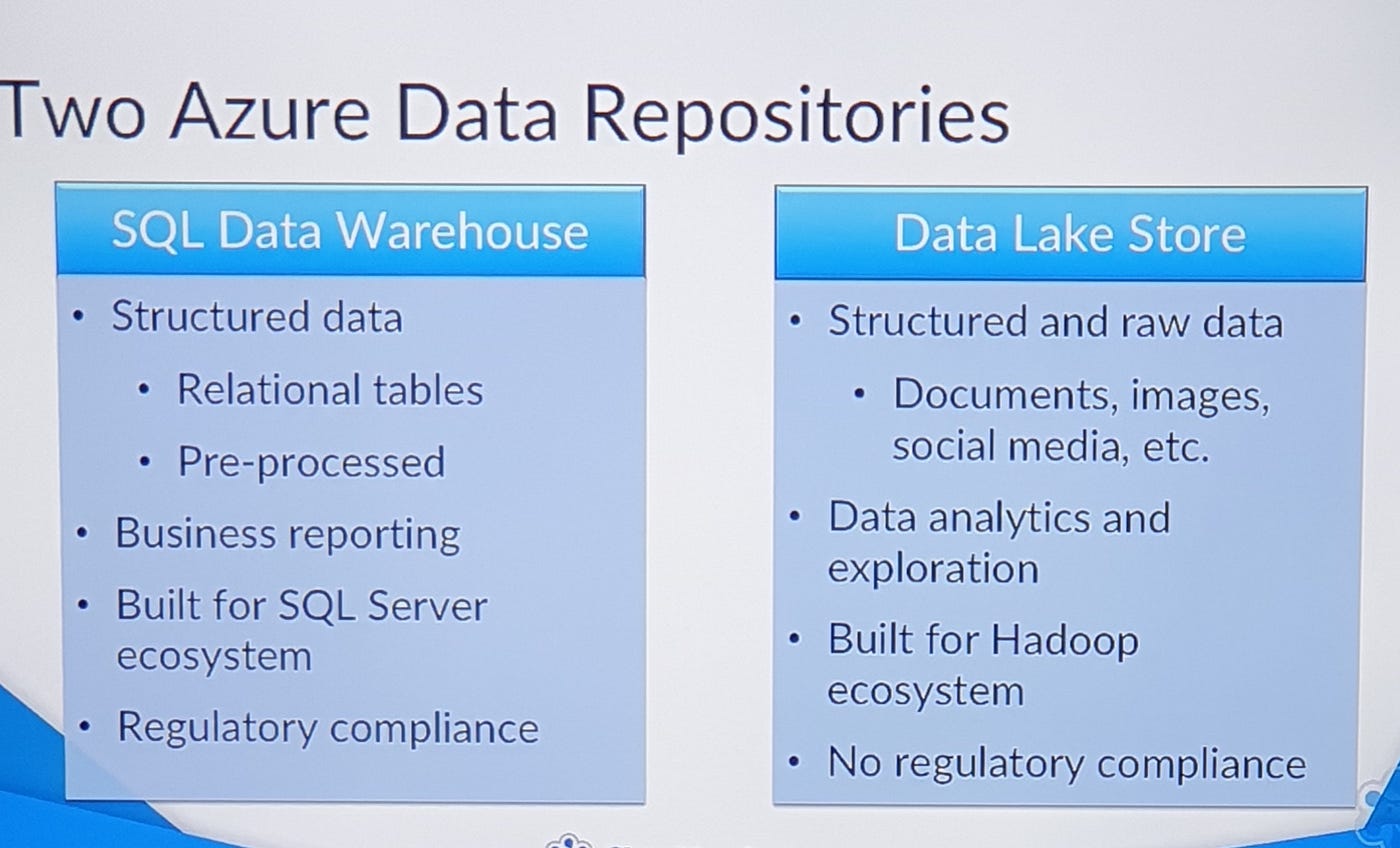
16. what is delta lake ?


No comments:
Post a Comment